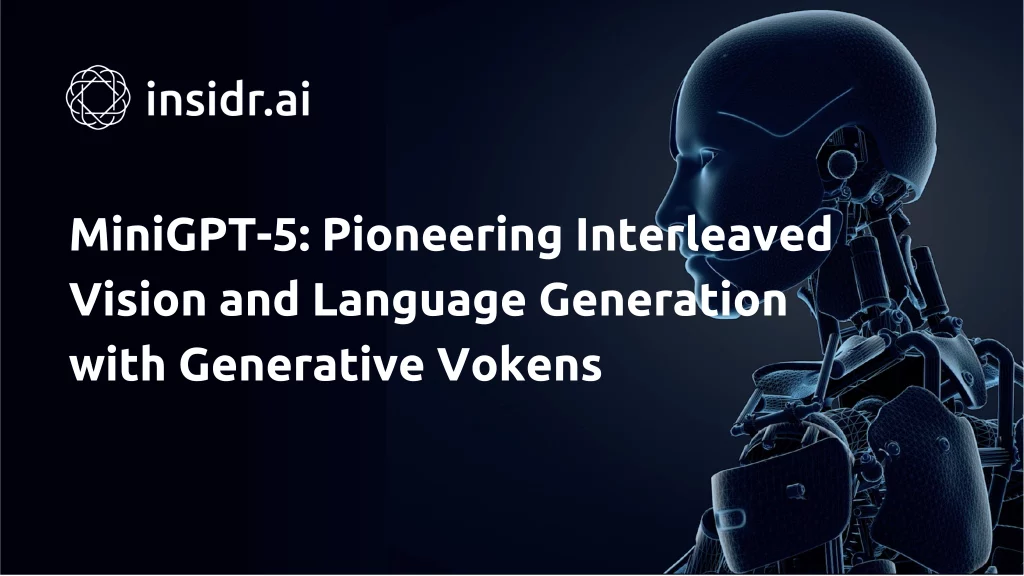
In recent years, the ascent of Large Language Models (LLMs) has captivated the AI community due to their transformative impact on Natural Language Processing (NLP).
These models have completely changed the game of text generation and comprehension.
However, despite the remarkable progress in text generation, producing images that seamlessly align with textual narratives has remained a formidable challenge.
To address this issue, a novel approach blending vision and language generation has emerged, employing the innovative concept of “generative vokens” to bridge the gap and achieve harmonious text-image outputs.
The Genesis of MiniGPT-5
At the core of MiniGPT-5 lies a two-stage training strategy, heavily focused on generating multimodal data without relying on exhaustive image descriptions.
Additionally, the model incorporates a classifier-free guidance system to enhance the efficacy of vokens for image generation.
In its initial phase, the MiniGPT-5 framework has exhibited impressive performance, surpassing the baseline Divter model trained on the MMDialog dataset.
Notably, it consistently outperforms the benchmark in human evaluations conducted on the VIST dataset, underlining its prowess across various metrics.
MiniGPT-5: What Is It?
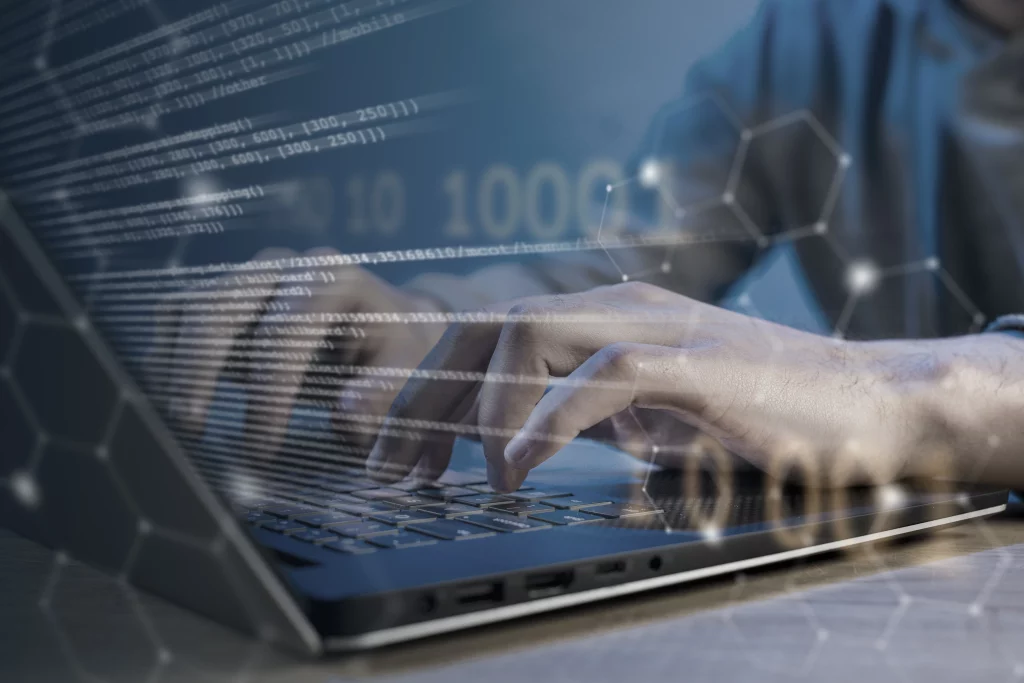
Recent advancements in LLM frameworks have paved the way for multimedia feature integration, a field gaining significant popularity due to its crucial role in a wide range of applications.
These applications span from cutting-edge content creation tools to advanced multimodal dialogue agents.
The ongoing research in language and vision models aims to enable the seamless generation of both textual and visual data.
The seamless generation of multimodal data has the potential to revolutionize interactions across domains, such as e-commerce, media, and virtual reality.
The primary objective is to empower models to synthesize, recognize, and respond consistently in both textual and visual modalities.
This endeavor is driven by the demand for more fluid, integrated, and interactive multimodal interactions in LLMs, ultimately resulting in alternating language and vision generation.
However, achieving integrated and interactive multimodal interactions in LLMs is a complex task riddled with several challenges:
- Current LLMs excel in text generation and processing text-image pairs but struggle to generate images.
- The development of vision and language models heavily relies on topic-focused data, making it challenging to align generated text with corresponding images.
- As LLM capabilities expand, memory requirements increase, particularly when handling downstream tasks.
The MiniGPT-5 framework, an interleaved language and vision generation technique that introduces the concept of “generative vokens,” aims to tackle these challenges.
This framework proposes a new approach to multimodal data generation by fusing Large Language Models with Stable Diffusion techniques, employing special visual tokens.
The two-stage training method employed by MiniGPT-5 emphasizes the importance of an initial stage devoid of descriptions, preparing the model to perform efficiently, even in scenarios with limited data.
What Sets MiniGPT-5 Apart
What distinguishes MiniGPT-5 from existing frameworks is that its generic stages don’t rely on domain-specific annotations.
To ensure the generated text and images are harmonious, MiniGPT-5 deploys a dual-loss strategy that enhances the framework’s use of classifier-free guidance and generative vokens.
MiniGPT-5 optimizes training efficiency and addresses memory constraints through its parameter-efficient fine-tuning strategy.
In summary, MiniGPT-5:
- Proposes a method using multimodal encoders, generative tokens, and Stable Diffusion techniques to generate interleaved language and visual outputs effectively.
- Introduces a dual-stage training strategy to generate description-free multimodal output, incorporating classifier-free guidance to enhance data quality.
MiniGPT-5: Method, Architecture, and Framework
To equip large language models with the capability for multimodal data generation, the MiniGPT-5 model introduces a framework that integrates text-to-image generation models and pretrained multimodal large language models.
The MiniGPT-5 framework introduces “generative vokens,” special visual tokens that address discrepancies across different domains, enabling training directly on raw images.
To enhance the quality of multimodal data generated by LLMs, the framework introduces a classifier-free strategy combined with an advanced two-stage training method.
Multimodal Input Stage
Recent developments in LLMs have unveiled their multimodal comprehension abilities, enabling them to process images as sequential input.
The MiniGPT-5 framework employs specially designed generative vokens to produce visual features, expanding LLMs’ ability to generate multimodal data.
Furthermore, the framework utilizes parameter-efficient fine-tuning techniques for multimodal output learning within the LLM framework.
Multimodal Encoding
The pretrained visual encoder in MiniGPT-5 transforms each input image into a feature, and text tokens are embedded as vectors.
The input prompt features are generated by concatenating these embeddings.
Incorporating Vokens in Large Language Models
Traditionally, the vocabulary of Large Language Models includes only textual tokens.
To bridge the gap between generative and traditional LLMs, the MiniGPT-5 framework introduces a set of special tokens as generative vokens into the LLM’s vocabulary.
These vokens’ hidden output state is harnessed for subsequent image generation, with the position of the vokens representing the insertion of interleaved images.
Parameter Efficient Fine Tuning (PEFT)
PEFT is a crucial concept in training LLMs, but its application in multimodal settings is still relatively unexplored.
MiniGPT-5 uses Parameter Efficient Fine Tuning over the MiniGPT-4 framework’s encoder to improve the model’s understanding of prompts and enhance performance in zero-shot or novel environments.
Multimodal Output Generation
To ensure accurate alignment between the generative model and the generative tokens, MiniGPT-5 introduces a compact mapping module to match dimensions.
It incorporates supervisory losses, including latent diffusion model loss and text space loss.
The latent diffusion supervisory loss aligns the visual features with the tokens directly, while the text space loss helps the model learn the correct token positions.
Since the generative vokens are guided by the images, MiniGPT-5 doesn’t require comprehensive image descriptions, enabling description-free learning.
Text Space Generation
MiniGPT-5 follows a casual language modeling method to jointly generate vokens and texts in the text space.
During training, vokens are appended to the ground truth image positions, and the model is trained to predict vokens during text generation.
Mapping Voken Features for Image Generation
Upon text space generation, the system synchronizes the concealed output state with the text-based conditional feature environment of the text-to-image generation model.
This process includes the integration of a feature mapping unit comprising a bipartite multilayer perceptron (MLP) model, an adaptable decoder feature sequence, and a quadruple-layer encoder-decoder transformer model.
Image Generation with Latent Diffusion Model (LDM)
To generate the required images during the denoising process, the framework uses mapping features as a conditional input.
The framework leverages a Latent Diffusion Model (LDM) for guidance, converting the ground truth image into a latent feature using a pre-trained VAE.
The latent noise feature is then obtained by adding some noise.
The comprehensive approach employed by MiniGPT-5 enables developers to achieve a coherent understanding and generation of both visual and textual elements.
It leverages specialized tokens, pre-trained models, and innovative training techniques.
MiniGPT-5: Training and Results
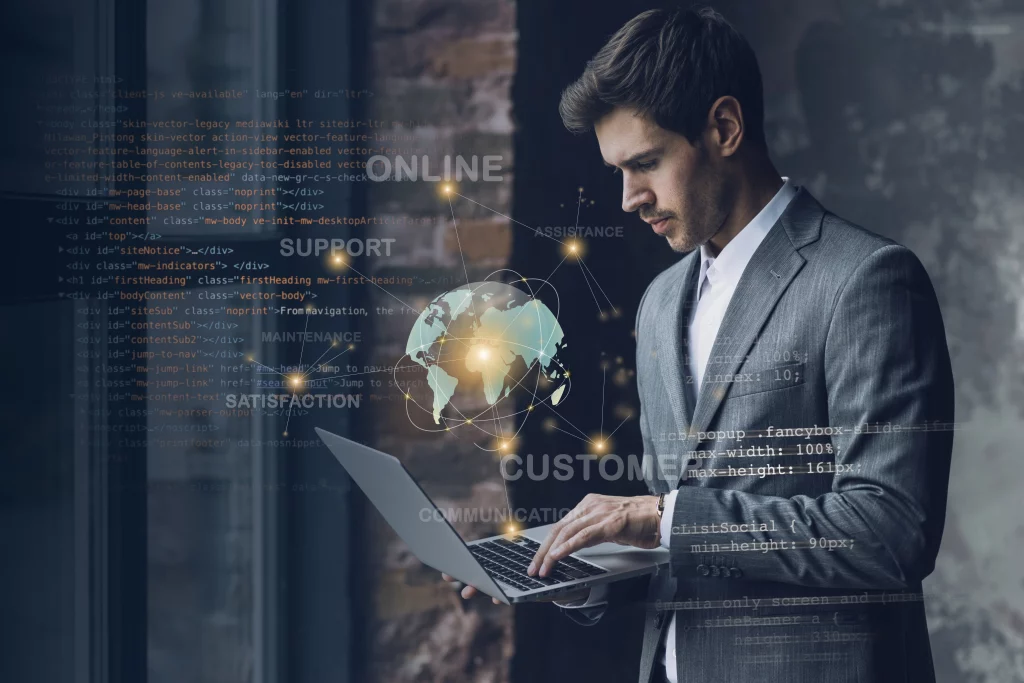
During the development of MiniGPT-5, the team encountered challenges when directly training on a limited interleaved text-and-image dataset.
This approach resulted in images with reduced quality and alignment issues, given the significant domain shift between image and text domains.
To overcome these challenges, the team adopted two distinct training strategies:
- Incorporating classifier-free guidance techniques to enhance the effectiveness of generative tokens during the diffusion process.
- A two-stage training strategy:
- Unimodal Alignment Stage (UAS)
- Multimodal Learning Stage (MLS)
The UAS initially aligns image generation features with voken features using single text-image pair datasets where each sample contains only one text and one image, typically an image caption.
Once the UAS is successful, the model can generate images for single text descriptions but struggles with interleaved language and vision generation.
To address this, the MiniGPT-5 framework is fine-tuned using PEFT parameters with interleaved vision-and-language datasets like VIST.
There are three tasks of construction during this stage:
- Text-Only Generation: Generates related text given the next image.
- Image-Only Generation: Generates the related image given the next text.
- Multimodal Generation: Produces text-image combinations based on the provided context..
MiniGPT-5: Benchmarks and Results
To comprehensively evaluate its performance in multimodal generation, the MiniGPT-5 development team compared it with other prominent baseline models, including Divter, GILL, and the Fine-Tuned Unimodal Generation Model.
The performance comparisons indicated that MiniGPT-5 outperforms these baselines.
The MiniGPT-5 framework recognizes that multimodal output may be contextually meaningful but may differ from ground reality.
To evaluate the model’s performance, it incorporates human assessments, considering three crucial perspectives:
- Language Continuity: Assessing whether the generated content seamlessly aligns with the provided context.
- Image Quality: Evaluating the relevance and clarity of the generated images.
- Multimodal Coherence: Determining whether the combined text-image output is in sync with the initial context.
VIST Final Step Evaluation
In the initial experiments, MiniGPT-5 aimed to generate corresponding images, yielding noteworthy results.
The figure above highlights the performance of MiniGPT-5 compared to the fine-tuned MiniGPT-4 framework using performance metrics like S-BERT, Rouge-L, and Meteor.
It indicates that the use of generative vokens does not negatively impact the framework’s performance in multimodal comprehension tasks.
The results show that MiniGPT-5 is capable of utilizing long-horizontal multimodal input prompts across a wide range of data to generate high-quality and coherent images without compromising the model’s original ability for multimodal comprehension.
The table above compares the performance of three frameworks on 5,000 samples for multimodal generation across Multimodal Coherence, Image Quality, and Language Continuity.
MiniGPT-5 outperforms the other two baseline models in more than 70% of cases.
Despite data limitations, the table below demonstrates the performance of MiniGPT-5 on the CC3M validation dataset for single image generation, outperforming the current state-of-the-art baseline GILL framework across all metrics.
VIST Final Step Evaluation
In the initial experiments, MiniGPT-5 aimed to generate corresponding images, yielding noteworthy results.
The figure above highlights the performance of MiniGPT-5 compared to the fine-tuned MiniGPT-4 framework using performance metrics like S-BERT, Rouge-L, and Meteor.
It indicates that the use of generative vokens does not negatively impact the framework’s performance in multimodal comprehension tasks.
The results show that MiniGPT-5 is capable of utilizing long-horizontal multimodal input prompts across a wide range of data to generate high-quality and coherent images without compromising the model’s original ability for multimodal comprehension.
The table above compares the performance of three frameworks on 5,000 samples for multimodal generation across Multimodal Coherence, Image Quality, and Language Continuity.
MiniGPT-5 outperforms the other two baseline models in more than 70% of cases.
Despite data limitations, the table below demonstrates the performance of MiniGPT-5 on the CC3M validation dataset for single image generation, outperforming the current state-of-the-art baseline GILL framework across all metrics.
MiniGPT-5 Conclusion
In this piece, we’ve delved into MiniGPT-5, an innovative algorithm that combines language and vision for generating content while introducing the concept of “generative vokens.”
This approach harnesses the capabilities of Large Language Models to produce multimodal data by aligning the extensive language model with a text-to-image generation framework.
We’ve examined the fundamental elements, structure, and outcomes of this framework, highlighting substantial enhancements in performance and efficiency when compared to established baseline models.
MiniGPT-5 aims to establish a new standard in the field of generating multimodal content and data, effectively addressing challenges that previous models encountered in tackling similar tasks.
Source
Discover More AI Tools
Every week, we introduce new AI tools and discuss news about artificial intelligence.
To discover new AI tools and stay up to date with newest tools available, click the button.
To subscribe to the newsletter and receive updates on AI, as well as a full list of 200+ AI tools, click here.