As technology continues to grow, understanding AI isn’t just for professionals—it’s something anyone can learn. We’re going to break down over 50 AI terms in a way that’s clear and easy to follow.
If you’re new to the world of AI or want to expand what you already know, these bite-sized explanations will give you the confidence to explore artificial intelligence in a whole new way.
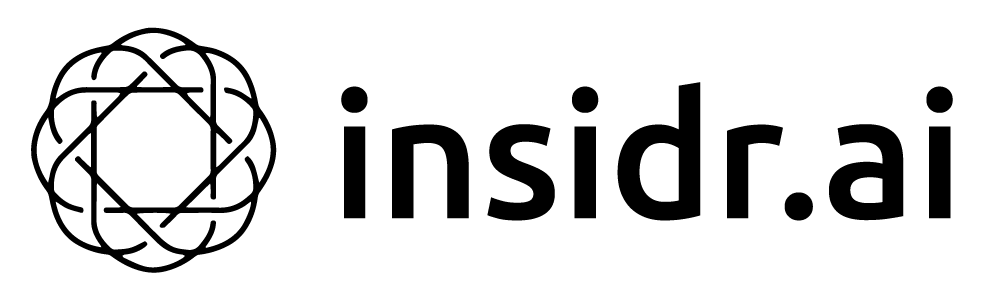
AI Solutions
AI-proof your business by integrating the right tools and systems for your needs. Discover solutions and products.
Artificial Intelligence (AI)
Artificial Intelligence refers to the ability of machines to perform tasks that ordinarily need human intelligence. These activities may include solving puzzles, deciphering language, and spotting patterns.
AI is not about robots taking over the world; it’s about making machines smart enough to assist us in everyday activities, from virtual assistants like Siri to recommendations on Netflix.
Artificial Superintelligence (ASI)
Artificial Superintelligence (ASI) represents a level of AI that surpasses human intelligence across all domains, including creativity, problem-solving, and social intelligence.
This hypothetical form of AI would not only exceed human cognitive abilities but could also potentially self-improve at an accelerated rate, leading to rapid advancements in technology and capabilities.
The concept of ASI raises significant questions about control, ethics, and the future of humanity, as it would represent an intelligence far beyond our own and might have profound implications for society and global dynamics.
Artificial Narrow Intelligence (ANI)
Artificial Narrow Intelligence (ANI), or weak AI, refers to AI systems that are built to handle specific tasks. These systems are good at what they’re programmed to do but don’t have the ability to think or adapt beyond those tasks.
A good example of ANI is a movie recommendation system that suggests what to watch next based on your past choices, or a spam filter that blocks unwanted emails. While ANI is effective at performing its job, it can’t apply what it knows to other tasks outside of its specific purpose.
AI System
An AI System is a complex setup that brings together different technologies to perform tasks that usually require human thinking. These systems mimic how humans learn, reason, solve problems, and make decisions.
For example, think of a voice assistant that can understand and respond to your commands. It uses several components: speech recognition to understand what you’re saying, natural language processing to figure out what it means, and a response generator to give you the right answer or action.
To work effectively, AI systems also need access to data or knowledge sources to make informed decisions and provide useful responses.
AI Model
An AI model is essentially a computer program designed to perform specific tasks by learning from data. It’s the brain of an AI system, taking in information and making sense of it to produce a result. The process of training a model involves feeding it lots of data so it can recognize patterns and features relevant to the task it’s meant to handle.
For example, if the task is recognizing animals in pictures, the model might be trained using thousands of labeled images of cats and dogs. Over time, it learns to tell the difference by spotting patterns in the images. Once trained, the model can look at a new picture and figure out if it’s a cat or a dog based on what it’s learned.
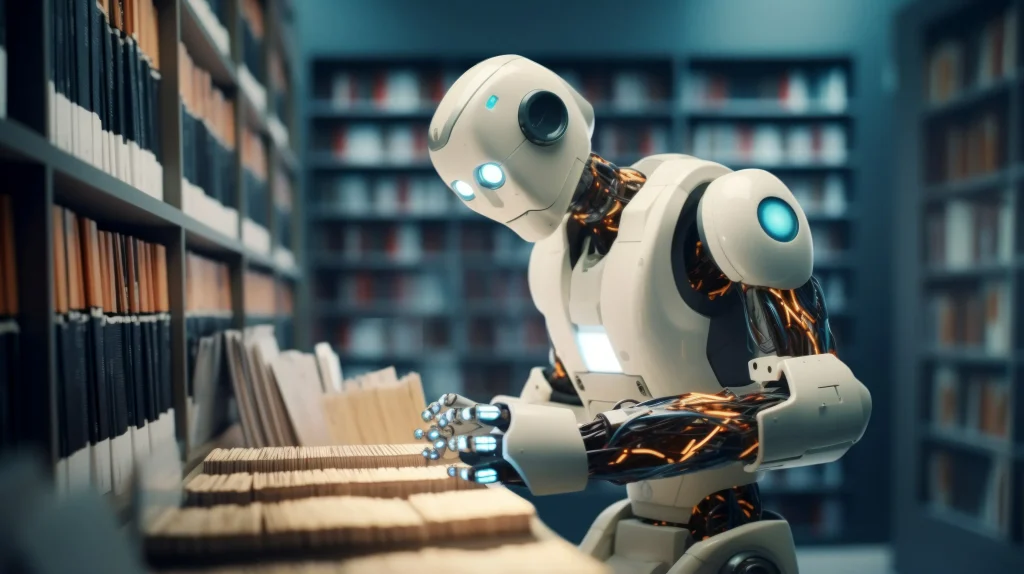
Artificial General Intelligence (AGI)
Artificial General Intelligence (AGI) refers to a form of AI that possesses the ability to understand, learn, and apply knowledge across a broad range of tasks, similar to human intelligence. Unlike current AI systems, which are specialized for specific tasks, AGI would be capable of generalizing knowledge and skills from one area to another.
For example, an AGI system could learn new skills or solve problems in various fields, from scientific research to creative endeavors, with the same level of competence as a human. Achieving AGI remains a long-term goal in AI research, as it involves creating machines that can perform any intellectual task that a human can.
Automation
Automation involves using technology to perform tasks that would otherwise require human intervention. In the context of AI, automation refers to systems that can execute repetitive tasks, make decisions, or process data with minimal human oversight.
Consider an automated email filtering system. It sorts incoming emails into different folders (e.g., inbox, spam, promotions) based on predefined rules and patterns learned from previous emails. This reduces the need for manual sorting and speeds up the process of managing emails.
Anomaly Detection
Anomaly Detection is the process of identifying unusual or unexpected patterns in data that deviate from the norm. It’s used to detect outliers or irregularities that might indicate problems or opportunities.
For instance, in cybersecurity, anomaly detection systems monitor network traffic for unusual activity that could signify a potential security breach. If a network usually has a certain volume of data transfer and suddenly experiences a spike, the system flags this as an anomaly, prompting further investigation.
Black Box
A Black Box in AI refers to a system whose internal workings are not transparent or understandable. Users can see the inputs and outputs, but the process that connects them is hidden. This lack of transparency can be problematic, especially when decisions made by the AI affect people’s lives.
For example, a deep learning model that predicts stock prices might be considered a Black Box because, while it produces predictions, it’s difficult to understand how it arrived at those predictions due to the complexity of the algorithms and the numerous factors involved.
Computer Vision
Computer Vision is a part of artificial intelligence that focuses on teaching machines to interpret and understand visual information, like images or videos, just like humans do.
It uses algorithms and models to process this visual data, helping machines recognize objects, spot patterns, and understand what’s happening in a scene. By combining techniques from image processing and machine learning, computer vision allows computers to “see” and make sense of the world.
Computer vision is used in many areas. In healthcare, it can analyze medical images to detect things like tumors. In retail, it helps track inventory by monitoring stock levels and identifying products on shelves. Self-driving cars also rely on computer vision to navigate roads, recognize pedestrians, road signs, and other vehicles.
Computer Systems
Computer systems include all of the hardware and software elements required to carry out computations. Physical components such as the central processing unit (CPU), RAM, storage devices, and input/output peripherals are referred to as hardware. The operating system, apps, and utility programs that let the hardware do certain tasks are examples of software.
Hardware supplies the physical resources required for processing and storing data, and software controls and guides these resources to carry out tasks. Computer systems function as one integrated entity.
A desktop computer system, for instance, consists of the hardware (the actual machine) and the software (the operating system) that controls hardware resources and enables users to execute programs like web browsers and word processors.
Computing Power
Computing Power refers to the capacity of a computer system to perform calculations and process data. It is often measured by metrics such as clock speed (in GHz), the number of cores, and processing capabilities (like FLOPS—floating-point operations per second).
Higher computing power enables a system to handle more complex tasks, execute processes faster, and manage larger volumes of data.
In practical applications, computing power is crucial for tasks that require significant processing, such as scientific simulations, big data analytics, and complex machine learning algorithms.
For instance, high-performance computing systems used for climate modeling can simulate large-scale weather patterns more accurately and quickly due to their substantial computing power.
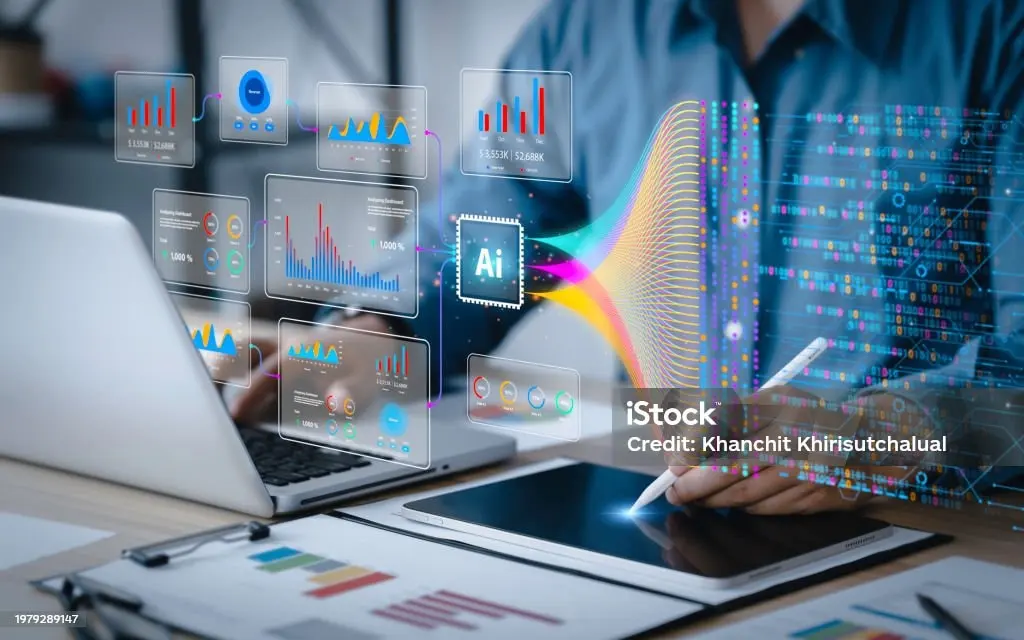
Constitutional AI
Constitutional AI involves training AI models with a set of guiding principles or rules, often referred to as a “constitution,” to ensure that their behavior aligns with human values and ethical standards.
This approach encourages AI systems to self-regulate and avoid harmful actions based on predefined norms, such as avoiding bias or misinformation.
For instance, a constitutional AI might be programmed to adhere to principles like fairness and accuracy, ensuring that its outputs are responsible and aligned with societal values.
Deep Learning
Deep Learning is a type of machine learning that uses neural networks with many layers. These layers allow a machine to process information in a way similar to how the human brain works.
Deep learning is particularly useful for tasks like image and speech recognition, where it can analyze vast amounts of data to find patterns and make decisions.
Data Augmentation
Data augmentation involves creating new data from existing data to improve a model’s performance. This can include techniques like flipping, rotating, or cropping images to generate more examples for training.
By augmenting data, we can help the model learn more effectively, especially when the original dataset is small. This technique is commonly used in image recognition tasks.
Embedding
Embedding is like giving meaning to data in a way that captures its essence and context. It is a way to turn words, images, or other information into a format that an AI model can understand.
For example, in natural language processing (NLP), embeddings help the model grasp the relationships between words—like knowing that “cat” and “kitten” are related. By using embeddings, AI can better understand the subtleties of language or recognize patterns in images, making it more effective in tasks like language translation or image recognition.
Ethical AI
Ethical AI is about creating and using AI in ways that align with moral values and social standards. It focuses on making sure AI systems are fair, transparent, and accountable. The goal is to ensure AI benefits everyone and doesn’t reinforce existing biases or inequalities.
Take facial recognition as an example. Ethical AI would ensure the technology works accurately for all demographic groups and doesn’t discriminate. It also involves thinking about privacy and making sure the system respects people’s rights and freedoms when it’s put to use.
Explainability
Explainability in AI means being able to understand how an AI system comes to its decisions. It’s about making the inner workings of AI clear and transparent to people, which is especially important in areas like healthcare or finance, where trust is crucial.
For example, if an AI model decides whether a loan should be approved or denied, explainability would show what factors, like income or credit score, influenced the decision. This transparency helps users see why a particular decision was made and ensures the AI is being fair and consistent.
Expert System
An Expert System is an AI application designed to emulate the decision-making abilities of a human expert. It uses a knowledge base of expert information and a set of rules to solve complex problems or make recommendations.
For example, a medical diagnostic expert system might be used to help doctors diagnose diseases. It uses a knowledge base of medical information and symptoms to suggest possible diagnoses based on patient data. While it doesn’t replace human expertise, it aids in decision-making by providing expert-level advice and insights.
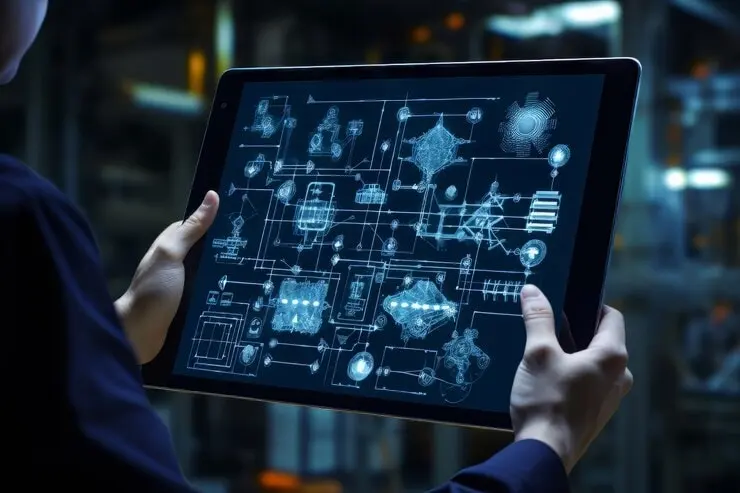
Few-Shot Learning
Few-Shot Learning allows a model to learn and perform tasks with very limited examples. This method is useful when there isn’t a lot of data available for a new task, as the model can generalize from just a few examples to understand and respond appropriately.
Suppose you have a model that needs to recognize a new type of flower with only a few images. Few-Shot Learning helps the model quickly adapt and identify similar flowers by generalizing from the limited examples provided.
Fine-Tuning
Fine-Tuning involves taking a pre-trained model and adjusting it for a specific task or type of data. This process refines the model’s abilities by continuing its training with a more focused dataset related to the desired application.
For instance, a language model trained on general text data can be fine-tuned with medical journals to specialize in medical terminology and concepts. This adaptation allows the model to generate or analyze text with a higher degree of accuracy in the medical field.
Foundation Model
A Foundation Model is an AI model trained on a broad range of unstructured data, such as text, images, or audio, to create a general-purpose base. This model isn’t designed for a single task but serves as a strong starting point that can be fine-tuned for specific applications.
It is like a generalist who knows a bit about everything; with additional training, this “generalist” can quickly become an expert in a particular field, like medical diagnosis or customer service chatbots.
Generative AI
Generative AI refers to technology that creates new content based on learned patterns from existing data. Instead of just analyzing or classifying data, generative AI produces novel data that resembles what it has been trained on.
For instance, a generative AI can create original pieces of music or artwork by learning from existing compositions or styles. It can generate unique images that mimic certain artistic styles or compose music that follows a specific genre’s patterns, demonstrating its creative capabilities.
Generative Adversarial Networks (GANs)
Generative Adversarial Networks (GANs) are a powerful tool in AI where two neural networks work against each other. One network, the generator, creates new data samples, while the other, the discriminator, evaluates these samples against real data to determine their authenticity. This adversarial process helps improve the quality of the generated data.
Imagine GANs being used to create realistic images. The generator might produce an image of a fictional landscape, while the discriminator assesses whether it looks real. Over time, this competition helps the generator create increasingly realistic images, which can be used for applications like designing virtual environments or enhancing photo quality.
Generative AI Models
Generative AI Models are a category of AI designed to create new and original content, such as text, images, or music, based on patterns learned from existing data. Unlike models that merely analyze or classify data, generative models actively produce new data that mimics the characteristics of the input data.
For example, generative models can create realistic images of fictional people or write coherent and contextually appropriate paragraphs of text. These models are used in various applications, including creative writing, art generation, and synthetic media production.
Graphics Processing Unit (GPU)
A Graphics Processing Unit (GPU) is a specialized processor designed to handle rendering graphics and performing parallel computations. Originally developed for rendering images and video in gaming and graphics applications, GPUs are now widely used in machine learning and AI due to their ability to process many tasks simultaneously.
GPUs excel at handling parallel tasks, making them ideal for training large machine learning models that involve processing large datasets.
For example, when training a deep learning model for image recognition, the GPU can simultaneously process multiple data points, accelerating the training process compared to traditional central processing units (CPUs).
Hallucination
In AI, a Hallucination is when a model generates outputs that don’t make logical sense or are factually incorrect. Imagine asking an AI to write a story, and it starts making up characters or events that never happened, or an image model produces pictures with bizarre, distorted elements.
Hallucinations can happen because the AI is trying too hard to provide an answer or create content, even when it lacks the correct information. It’s a reminder that while AI can be impressive, it’s not always perfect!
Image-to-Image
Image-to-Image is a process where an AI takes one image and transforms it into another, while keeping some of its core features.
For example, you could give an AI a photo of a car, and it might create a new image that shows the same car but in a different color or style. This technique is useful for applications like enhancing photos, adding artistic effects, or even helping to fill in missing details in an image.
Inference
Inference is like an AI model putting its learning into action. After being trained on a lot of data, the model uses that knowledge to generate outputs or make predictions.
For instance, once an AI model learns to recognize cats in images, it uses inference to identify a cat in a new picture it hasn’t seen before. It’s the step where all the training pays off and the AI does what it was built to do!
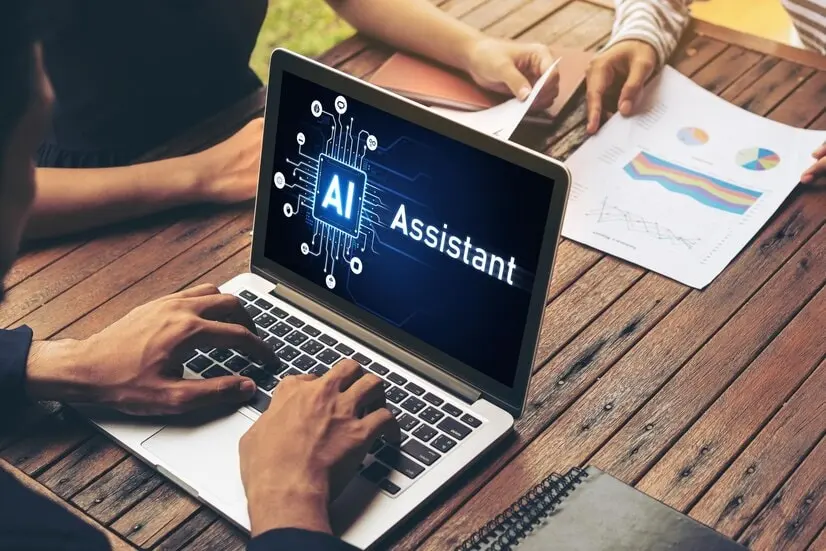
Large Language Model (LLM)
A Large Language Model (LLM) is a type of AI, like OpenAI’s GPT, that excels at understanding and generating human language. These models learn from vast amounts of text data to recognize patterns, relationships, and context in language, allowing them to perform tasks like writing articles, answering questions, or even having conversations.
It is like a supercharged version of a predictive text feature on your phone, but much more powerful and capable of understanding complex language nuances.
Low-Code/No-Code
Low-Code and No-Code platforms are tools designed to simplify the process of building applications by reducing the amount of coding required. Low-Code platforms offer a visual development environment with pre-built components and minimal coding, making it accessible to users with basic programming knowledge.
No-Code platforms go a step further by enabling users to create applications entirely through graphical interfaces and drag-and-drop features, without writing any code.
Machine Learning (ML)
Machine Learning is a subset of AI that enables machines to learn from data. Instead of being explicitly programmed to perform a task, a machine learning model analyzes patterns in the data and makes decisions based on what it has learned.
For example, ML helps your email app filter out spam by learning from previous emails you marked as spam.
Modality
Modality refers to the type of data that an AI model works with. This could be anything from text, images, and audio to video.
Different modalities require different types of processing. For example, understanding spoken language (audio) is quite different from interpreting a photograph (image). Multimodal AI systems can handle multiple types of data, making them versatile and powerful.
Multimodal AI
Multimodal is an AI system that can comprehend and utilize the data from multiple types of data such as text, images, and audio in myriads of tasks or decisions it is making. This capability allows the AI to understand and generate insights based on a richer and more comprehensive understanding of the data.
For instance, a multimodal AI system can go through different video scenes and can convert not only the visual component but also the spoken audio information into more precise descriptions or summaries. By blending the information of different data types, this AI can better handle diverse and multiple inputs, thus this improves its ability and makes it more useful overall.
Natural Language Processing (NLP)
Natural Language Processing (NLP) is a field in artificial intelligence (AI) that focuses on how computers can understand and interact with human language. Imagine teaching a computer to read, write, and even talk like a person.
NLP helps machines interpret and respond to text or spoken words in a way that feels natural to us. This is important for tasks like translating languages, understanding voice commands, and even answering questions in a chat.
Natural Language Processing tasks can range from simple ones, like recognizing the words in a sentence, to more complex ones, like understanding the meaning behind those words. For example, when you ask your phone to play a song, it uses NLP to understand your request and find the right song. NLP is everywhere in our daily lives, making our interactions with technology smoother and more intuitive.
Neural Networks
Neural Networks are the backbone of deep learning. They are made up of layers of nodes (like neurons in the brain) that process information.
Each node takes in data, processes it, and passes it on to the next layer. Over time, the network learns to make accurate predictions or decisions by adjusting the connections between nodes.
Neural Network Architecture: This refers to the structure of a neural network, including how many layers it has and how the nodes are connected. A well-designed architecture can greatly improve the performance of a deep learning model.
Differences Between Artificial Neural Networks and the Human Brain’s Structure: While neural networks are inspired by the human brain, they are much simpler. The human brain has billions of neurons connected in complex ways, while artificial neural networks have far fewer nodes and simpler connections.
Natural Language Understanding (NLU)
Natural Language Understanding (NLU) is a crucial part of how computers and AI systems interpret human language. When you interact with a digital assistant or chatbot, NLU processes your input to grasp not only the words you use but also the meaning behind them. This involves recognizing the intent of your message, extracting relevant details, and understanding the context.
Imagine you’re using a virtual assistant and you say, “I need to book a flight to New York next week.” NLU breaks this down to understand that you want to make a flight reservation and that the destination is New York. It also notes that the timing is for the following week.
The system then uses this understanding to take appropriate actions, such as searching for flight options and suggesting dates. NLU enables the system to handle various nuances in your language, such as slang or complex sentences, to provide a helpful response.
Natural Language Generation (NLG)
Natural Language Generation (NLG) is about creating text from data. It’s the technology that allows a system to take structured information and transform it into human-readable text. This process involves assembling sentences that are coherent and contextually appropriate based on the data provided.
Imagine a financial report generated by a software program. The program might receive raw data on sales, expenses, and profits. Using NLG, it can produce a report that reads something like, “The company saw a 10% increase in revenue this quarter, driven by higher sales in the electronics segment.” This summary is crafted to be clear and informative, making complex data more accessible and understandable for users.
Open Source
Open Source refers to software whose source code is publicly available for anyone to view, modify, and distribute. Open-source software is typically developed collaboratively by a community of developers who contribute to improving and expanding the software.
The open-source model promotes transparency and innovation, allowing users to adapt the software to meet their specific needs.
Open-source projects often have extensive documentation and community support, which facilitates learning and collaboration. Popular examples include the Linux operating system and the Apache web server. These projects benefit from contributions by developers worldwide, leading to continuous improvement and rapid problem-solving.
Perplexity
Perplexity is a way to measure how well a language model is doing its job. It indicates how confident the model is when predicting the next word in a sequence.
A lower perplexity score means the model is better at understanding and generating coherent text, while a higher score suggests it may struggle to make sense of the language or context.
Prompt
A Prompt is the input or query you give to an AI model to guide what it should do next. It’s like asking a question or giving a direction.
For example, you might provide a text description to an AI image generator to create a picture or ask a chatbot to explain a concept. The prompt provides the starting point for the AI to generate a relevant response or output.
Reinforcement Learning from Human Feedback (RLHF)
Reinforcement Learning from Human Feedback (RLHF) is a method where AI models learn from human guidance to improve their performance.
For example, a human might provide feedback on the quality of the AI’s responses in a conversation, and the model learns from this to produce better answers next time. This technique is especially useful in areas where human judgment is essential, like understanding complex language or making ethical decisions.
Responsible AI
Responsible AI refers to the practice of developing and deploying AI systems in ways that are ethical, transparent, and aligned with societal values. It involves ensuring that AI systems are designed and used in ways that respect privacy, fairness, and accountability.
Responsible AI might include implementing safeguards to prevent biased decision-making, ensuring data protection, and providing clear explanations of how AI decisions are made. The goal is to create AI systems that contribute positively to society and do not harm individuals or groups.
Reinforcement Learning
Reinforcement learning is a method where a model learns through trial and error, receiving rewards or penalties based on its actions.
This approach is often used in areas like gaming or robotics, where the model must make a series of decisions to achieve a goal. Over time, the model learns to maximize rewards by refining its strategy.
Retrieval Augmented Generation (RAG)
Retrieval Augmented Generation (RAG) is a technique that combines retrieving information from a database with generating text based on that information. The model first searches for relevant data and then uses it to create a detailed response or generate content.
For instance, if you query a RAG system about the benefits of a certain technology, it will first pull up relevant information from a knowledge base. It then synthesizes this information into a coherent and informative answer, ensuring that the response is both accurate and contextually rich.
Sentiment Analysis
Sentiment Analysis involves evaluating the emotional tone of a text to determine whether it’s positive, negative, or neutral. This technique helps in understanding the underlying feelings expressed in reviews, social media posts, or any written communication.
Businesses often use sentiment analysis to gauge customer opinions. For example, if a company reviews social media mentions of its new product, sentiment analysis can reveal overall customer satisfaction.
If many posts express excitement and praise, the company can infer that the product is well-received. Conversely, if the analysis highlights a lot of complaints or negative feedback, it can indicate areas needing improvement.
Singularity
Singularity refers to a hypothetical future point where artificial intelligence surpasses human intelligence, leading to rapid and unpredictable advancements in technology. At this stage, AI could potentially drive transformative changes across all areas of life, creating scenarios that are difficult to predict.
The concept of singularity raises questions about how to manage and align such powerful AI systems with human values and goals.
Speech Recognition
Speech Recognition technology converts spoken language into text. It’s the technology that allows you to speak to your device and have it transcribe your words. This technology is crucial for applications like voice assistants, transcription services, and hands-free controls.
When you use voice commands to ask your smart speaker to play a song or dictate a message, speech recognition is at work. The system listens to your speech, processes it to understand the content, and then transcribes or acts upon it. This makes interacting with technology more intuitive and accessible, especially for tasks that are easier to speak than to type.
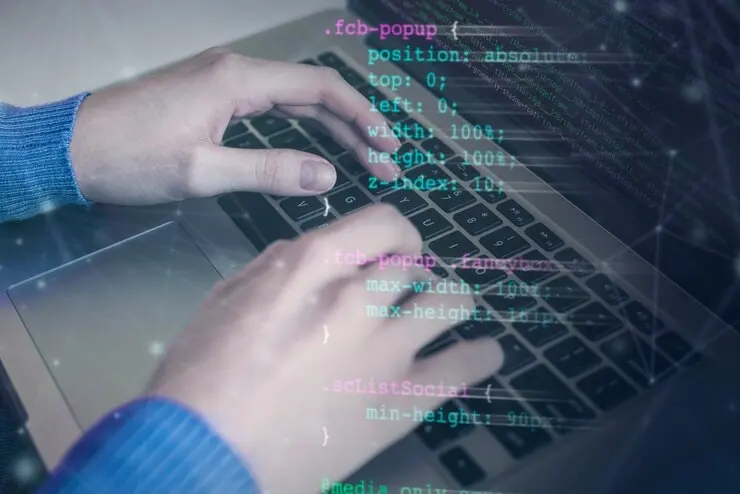
Steerability/Steerable AI
Steerability, or Steerable AI, refers to the ability of an AI system to be directed or guided in its behavior and outputs based on user preferences or instructions. This involves designing AI models that can be controlled or adjusted to meet specific requirements or constraints.
For example, a steerable AI system might allow users to fine-tune the style or tone of generated text or control the focus of a recommendation engine to align with particular interests. Steerability enhances the flexibility and usability of AI systems, making them more adaptable to diverse needs and applications.
Structured Data vs. Unstructured Data
Structured data is organized in a clear, predictable format, like a spreadsheet with rows and columns. This type of data is easy for machines to analyze.
Unstructured data, on the other hand, doesn’t follow a specific format. Examples include text, images, and videos. While more challenging to process, unstructured data often contains richer information and is increasingly important in AI applications.
Supervised Learning Algorithm
A supervised learning algorithm learns from labeled data. This means that each piece of training data comes with the correct answer, allowing the model to learn by example.
For instance, if you’re teaching a model to distinguish between apples and oranges, you would provide labeled images of both fruits. The model then learns to classify new, unlabeled images correctly.
Training Data
Training data is the information used to teach a machine learning model how to perform a specific task. The quality and quantity of training data directly impact the model’s accuracy.
For example, if you want a model to recognize cats in photos, you would feed it thousands of images of cats so it can learn to identify common features.
Text or Voice Commands
Text or Voice Commands are methods for instructing devices or applications to perform specific tasks. With text commands, you enter instructions through typing, such as searching for a document or launching an app on your computer.
Voice commands allow you to give instructions verbally, such as asking a smart speaker to set a reminder or check the weather.
Transformer
The Transformer is a key architecture in modern AI that excels at processing sequences of data, such as sentences. It uses attention mechanisms to focus on different parts of a sequence, helping it understand the context and relationships between words more effectively.
Transformers are integral to models like GPT. They allow the model to consider the entire context of a sentence when generating or interpreting text. This capability ensures that the responses are coherent and contextually appropriate, making the Transformer architecture crucial for advanced language tasks.
Tensor Processing Units (TPUs)
Tensor Processing Units (TPUs) are custom-built processors developed by Google specifically for accelerating machine learning tasks. TPUs are designed to handle tensor computations, which are central to many AI and deep learning models. They provide high performance and efficiency by optimizing matrix operations and parallel processing.
TPUs are particularly useful for training and running large-scale machine learning models. They can perform vast numbers of mathematical operations required for deep learning tasks more efficiently than general-purpose CPUs or GPUs.
For instance, TPUs are used in Google’s data centers to power various AI services, including search algorithms and recommendation systems.
Token
A Token is the smallest piece of data that an AI model processes. In text, a token could be a single word or even a part of a word. In an image, it might be a pixel or a small group of pixels. Tokens help break down complex data into smaller, manageable parts that the model can analyze and learn from.
Turing Test
The Turing Test is a benchmark for determining whether a machine exhibits intelligent behavior indistinguishable from that of a human. Proposed by Alan Turing, the test involves a human evaluator interacting with both a machine and a human without knowing which is which.
If the evaluator cannot reliably tell the machine from the human based on their responses, the machine is said to have passed the Turing Test. This test is used to assess the effectiveness of AI in mimicking human-like conversation and behavior.
Unsupervised Learning Algorithm
Unsupervised learning algorithms work with unlabeled data, meaning the model must find patterns or structures on its own. Instead of learning from examples, the model groups similar data points together.
This is useful for tasks like clustering customers based on purchasing behavior, where the goal is to find hidden patterns without predefined categories.
Vector
A Vector is a mathematical way of representing a token to help AI models understand its meaning in context. Imagine turning words or images into a set of numbers that capture their meaning, so the AI can work with them more effectively.
Vectors are crucial for tasks like finding similarities between different pieces of data or identifying patterns in complex datasets.
Zero-Shot Learning
Zero-Shot Learning enables a model to handle tasks or classify data it has never seen during training. Instead of relying on specific examples, the model uses its general knowledge and understanding of language or concepts to make predictions or solve problems.
For instance, if a model trained on general animal data encounters a new species it hasn’t been specifically trained on, it can still identify it based on its broader understanding of animal characteristics and classifications.
Discover More AI Tools
Join the free AI community to get free AI resources, join discussion, and learn how to use AI.
To subscribe to the newsletter and receive updates on AI, as well as a full list of 500+ AI tools, click here.