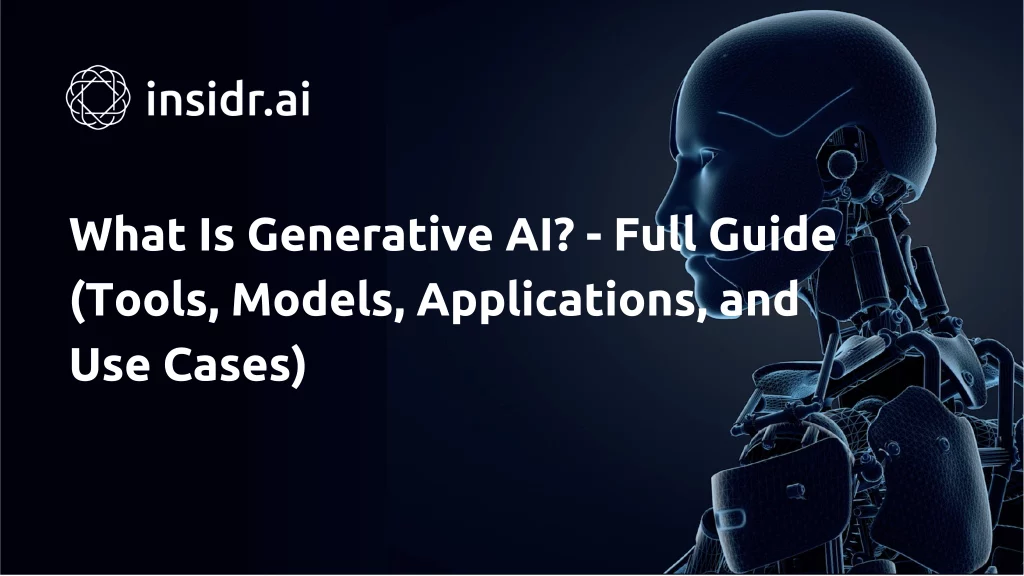
Imagine a world where content creation, from blog posts to music and even realistic images, can be generated at the push of a button, with the help of artificial intelligence. Welcome to the age of generative AI!
This rapidly evolving technology holds the potential to revolutionize industries and redefine the way we work and live.
In this blog post, we’ll delve into the world of generative AI, exploring its components, models, tools, real-world applications, and the challenges we need to address as it continues to advance.
So, let’s embark on a fascinating journey into the realm of generative AI.
Key Takeaways
- Generative AI is a powerful technology combining various algorithms to generate content such as text, images and audio.
- It involves deep learning models like GANs, VAEs and transformers. With open source & commercial tools available for different industries.
- Industry adoption of generative AI can lead to cost reduction & improved performance while ensuring ethical use through transparency & best practices.
Understanding Generative AI: From Theory to Practice
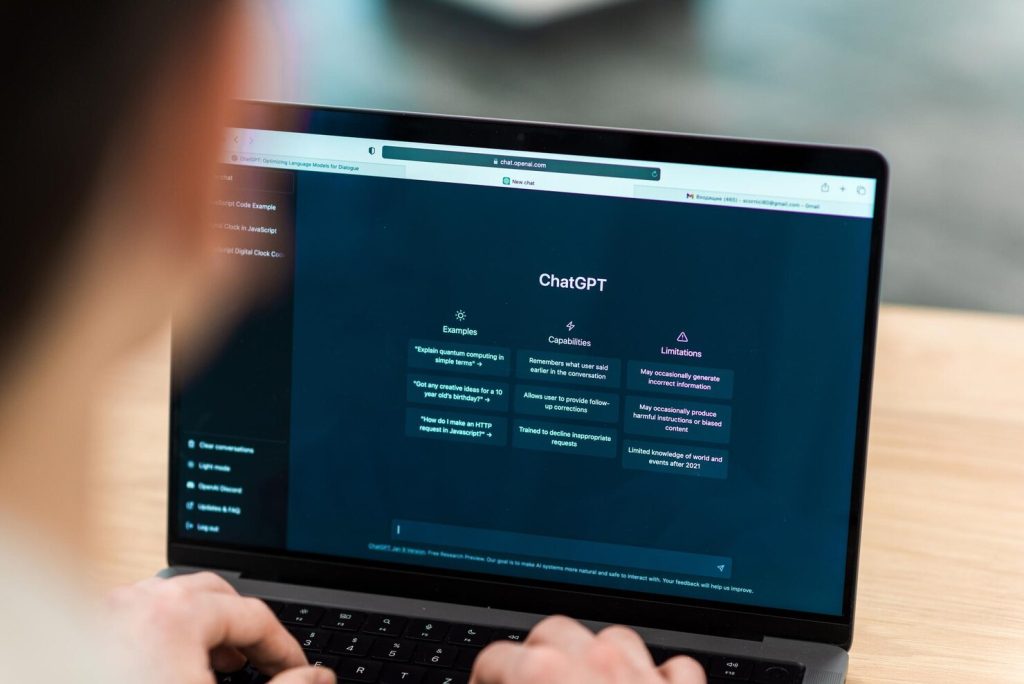
The concept of generative AI dates back to the 1960s, when Joseph Weizenbaum created the Eliza chatbot, which used training data to learn and generate responses.
Fast forward to today, and generative AI models have come a long way, combining various AI algorithms to create and manipulate content like:
The introduction of techniques like recurrent neural networks, deep learning, and generative adversarial networks (GANs) in recent years has further propelled the growth and development of generative AI.
Generative AI fundamentally uses deep learning models to produce new content from pre-existing data, with its utility stretching across multiple industries and harboring the ability to transform our work and life. But what is the working mechanism of generative AI, and what are its integral components?
How Generative AI Works
Utilizing neural networks and algorithms, generative AI discerns patterns within pre-existing data and creates statistically likely outputs like text, images, audio, or video.
A variety of natural language processing techniques are employed to turn raw characters into sentences, parts of speech, entities, and actions, which are then represented as vectors using various encoding techniques. Foundation models like GPT-3 and Stable Diffusion serve as a starting point for AI systems that can perform a wide range of tasks.
These generative AI models employ various machine learning approaches, including unsupervised or semi-supervised learning, for their training. With the latest advances in large language model (LLM) research, the industry has expanded its applications to include images, sounds, proteins, DNA, drugs, and even 3D designs.
Key Components of Generative AI
Generative AI’s main components encompass deep learning models such as GANs, variational autoencoders (VAEs), and transformers.
GANs, for instance, were introduced by Ian Goodfellow in 2014 and offer a unique approach to creating and evaluating content by pitting two neural networks against each other, resulting in realistic images, videos, and audio of real people.
Supervised learning techniques are also utilized in generative AI for customization and zero-shot learning.
With the continuous growth in the field, the development of new generative models and techniques like diffusion models and neural radiance fields is pushing the frontier of generative AI’s capabilities.
The Evolution of Generative AI Models
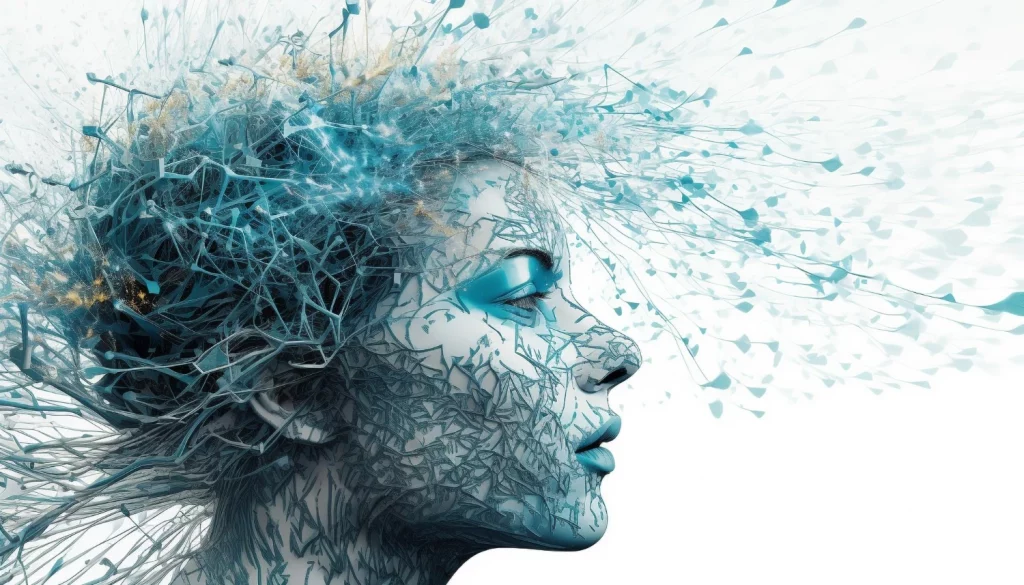
Over time, generative AI models have transformed from being smaller, specialized models into larger and more versatile ones, typified by GPT-3.
This shift has been primarily driven by the need to improve performance and capabilities, with larger models often yielding better results. Evidence has recently emerged which suggests that it is possible for smaller models trained on more specific data to outperform larger, general-purpose models. This highlights the importance of accuracy over scale..
Notable generative AI models include:
- Large language models like GPT-3, which are popular for their ability to perform a wide range of tasks, from content generation to conversational AI
- Diffusion models
- Domain-specific models
These models have been developed to cater to specific industries or use cases.
👉 Read more: Best AI Chatbots
Large Language Models
Large language models, such as GPT-3, have gained popularity due to their impressive capabilities and wide-ranging applications. These models can generate content like text, images, and audio, making them suitable for various tasks in marketing, entertainment, and design.
For example, ChatGPT by OpenAI uses GPT-3 to generate an essay based on a short text input.
Other chatbots such as Bing Chat, Bard, and LLaMA have been developed to use large language models. AI art systems like Stable Diffusion, Midjourney, and DALL-E have also been created to convert text to image. These advanced models showcase the versatility and potential of large language models in generative AI.
Other Notable Generative Models
Apart from large language models, there are other notable generative models like diffusion models, which, although they take longer to train, can have hundreds or even an infinite number of layers, making them ideal for building high-quality generative AI models.
Variational autoencoders (VAEs) are another popular type of deep learning model that was among the first to gain traction in creating realistic images and speech.
These deep generative models, along with many generative AI models like GANs, auto-regressive models, and flow-based models, continue to advance the field of generative AI, catering to a wide range of industries and use cases.
Tools and Platforms for Implementing Generative AI
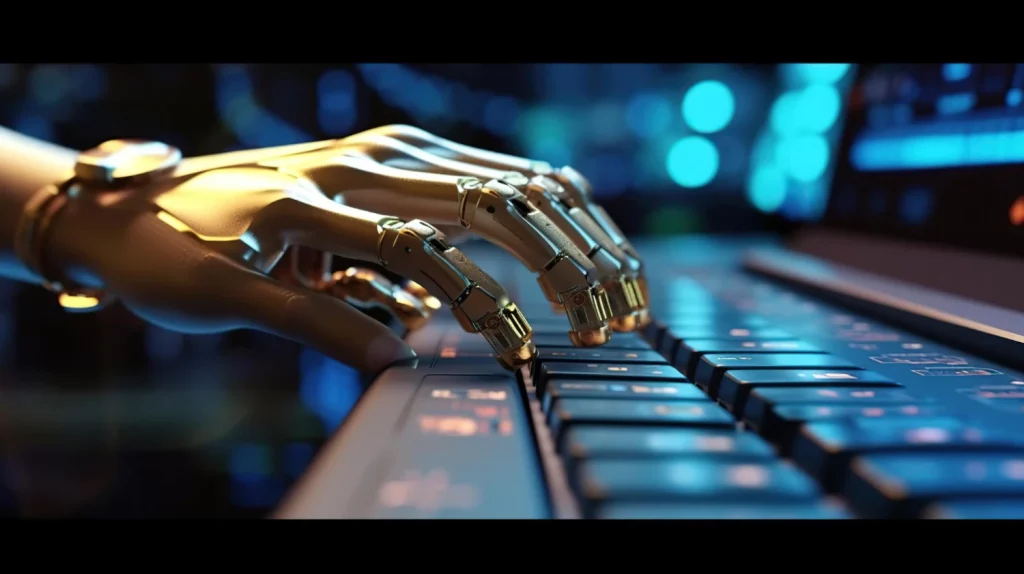
A variety of tools and platforms are available for implementing generative AI, ranging from open-source libraries like TensorFlow, PyTorch, and GPT-3, to commercial solutions provided by major tech companies like Google Cloud AI, Amazon SageMaker, and Microsoft Azure.
Given the availability of both open-source and commercial options, one can select the solution that aligns most closely with their needs and requirements.
Keep this prompt formula in mind when you use ChatGPT to get the results you seek.
Popular Generative AI Tools
Some of the most popular generative AI tools include:
- OpenAI’s ChatGPT: a versatile tool capable of creating content such as essays from brief text inputs
- Google’s Dall-E: associates words with visuals and is trained on an extensive collection of images and text descriptions
- NVIDIA’s AI Playground: a platform for exploring and experimenting with AI-generated content
These tools offer a range of capabilities for generating creative and unique content using artificial intelligence.
Other popular generative AI tools include:
- GPT-4
- AlphaCode
- GitHub Copilot
- Bard
- Cohere Generate
- Claude
- Synthesia
These tools showcase the variety of options available for different applications and industries.
Choosing the Right Tool for Your Needs
Selecting the perfect tool for your needs involves considering the application, the industry, and the level of customization required.
It’s essential to research and compare different tools in the market, checking out reviews, getting feedback from other users, and comparing features and functionalities.
In selecting a tool that integrates well with other necessary tools, it’s crucial to ensure compatibility and a straightforward integration process.
Additionally, focus on the tool’s user-friendliness and straightforward interface, so you can seamlessly incorporate generative AI into your workflows.
Real-World Applications of Generative AI Across Industries
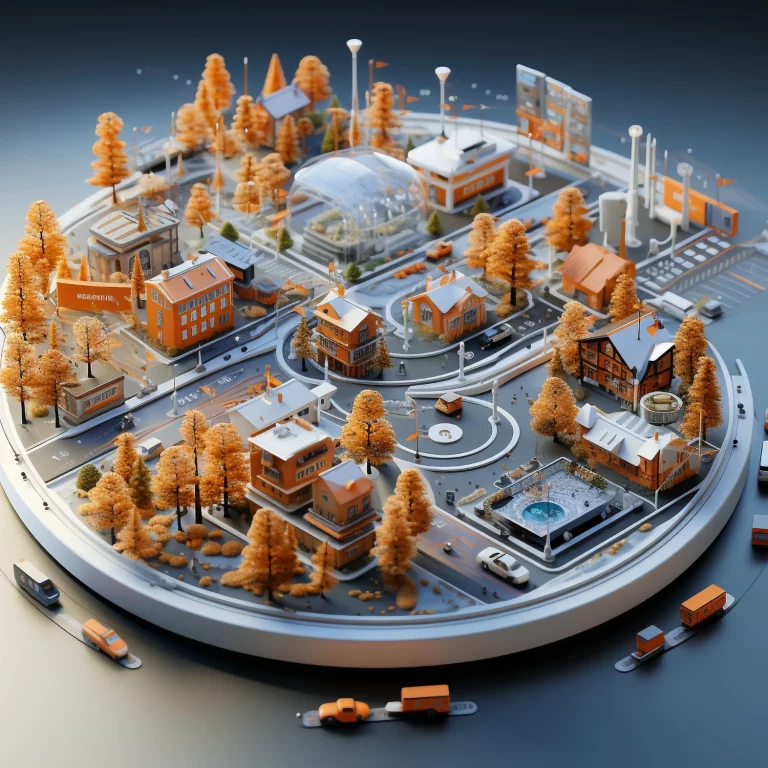
Generative AI boasts a multitude of real-world applications across diverse industries, including:
- Creative content creation
- Synthetic data production for AI model training
- Transportation
- Natural sciences
- Entertainment
These applications showcase the wide-ranging impact of generative AI on our daily lives.
Creative Content Generation
Creative content generation encompasses:
It has applications in marketing, entertainment, and design. For instance, generative AI can be used to generate marketing copy and personalized ads.
Predictions state that by 2025, 30% of outbound marketing messages from large organizations will be synthetically created.
In the pharmaceutical industry, generative AI is expected to significantly reduce costs and time spent on drug discovery, with projections indicating that around 30% of new drugs and materials will be created using generative AI by 2025.
Synthetic Data Generation
Synthetic data generation involves creating realistic datasets for training AI models, particularly in industries where data privacy or scarcity is a concern.
The process generates data that isn’t based on real-world data, helping to protect data privacy and reduce the cost and time associated with collecting and labeling real-world data.
Generative AI has found great application in various industries where generative AI work is transforming processes, including:
- Manufacturing
- Automotive
- Aerospace
- Defense
It is used for generative design, which allows engineers to create designs meeting predetermined criteria such as performance or materials/manufacturing methods requirements.
Navigating the Challenges of Generative AI
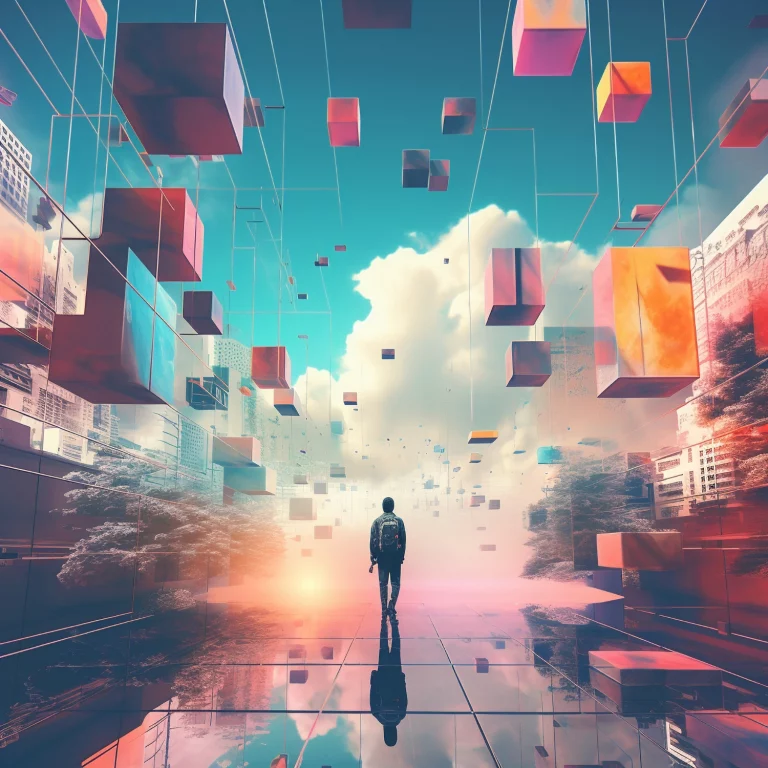
While generative AI offers numerous benefits and applications, it also comes with its fair share of challenges, including addressing bias and ethical concerns, as well as overcoming implementation hurdles.
Navigating these challenges and finding solutions adhering to best practices and industry standards is vital to ensure the responsible and effective use of generative AI.
Addressing Bias and Ethical Concerns
Generative AI systems can inadvertently introduce bias and raise ethical concerns, such as privacy and surveillance, discrimination, and the reinforcement of existing biases. To address these issues, it’s essential to promote transparency, due diligence, and the development of best practices for responsible use.
Another concern is the potential misuse or abuse of generative AI, such as creating fake news, deepfakes, or committing cybercrime. Ensuring the responsible use of generative AI requires a combination of technical safeguards, education, and ethical guidelines to mitigate these risks.
Overcoming Implementation Hurdles
Implementation of generative AI can present challenges, such as resource constraints, technology issues, human resource limitations, conflicting priorities, and pushback from stakeholders.
To overcome these hurdles, it’s important to select the right tools, models, and strategies for specific use cases and ensure proper integration with existing workflows and systems.
When integrating generative AI tools and models, consider their compatibility with existing systems, the potential for scalability, and the ease of integration.
By addressing these challenges and focusing on seamless implementation, businesses and organizations can harness the full potential of generative AI.
Looking Ahead: Predictions and Trends for Generative AI's Future
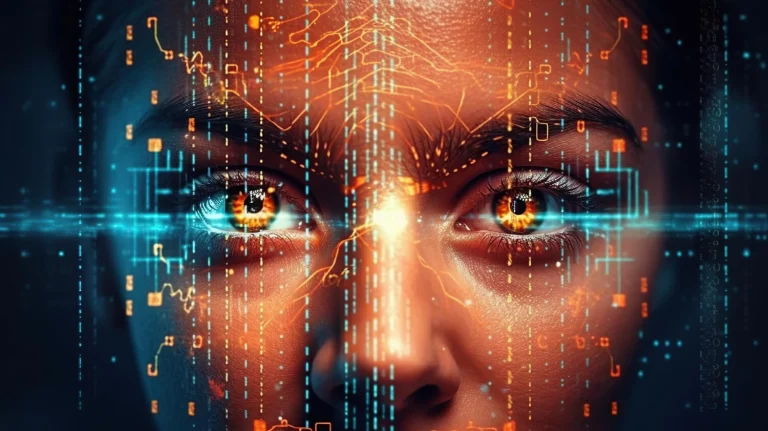
With the continuous advancement of generative AI, it is anticipated that industry adoption will rise and AI models and capabilities will markedly improve. The potential benefits of industry adoption include:
- Revenue growth
- Cost reduction
- Productivity improvement
- Risk management
These benefits can lead to a competitive advantage for early adopters.
Future advancements in generative AI models will likely focus on enhancing performance, versatility, and domain-specific capabilities, while addressing ethical concerns and limitations.
As technology progresses, the potential for generative AI to be utilized in various industries will only increase, paving the way for innovative solutions and applications.
Industry Adoption and Impact
The driving forces behind industry adoption of generative AI include the potential for revenue growth, cost reduction, productivity improvement, and risk management.
Early adopters of generative AI can gain a competitive edge by harnessing its capabilities for applications such as 3D modeling, product design, drug development, digital twins, supply chains, and business processes.
However, industry adoption also presents challenges, such as the need for significant resources, disruption to existing business models, and potential misuse of AI-generated content.
As generative AI continues to evolve and improve, businesses will need to adapt and overcome these challenges to stay competitive and benefit from the technology’s potential.
Advances in Generative AI Models
Generative AI models are constantly evolving, with advancements focusing on boosting performance, versatility, and domain-specific capabilities.
For example, optimizing a generative ai model to more effectively capture intricate data patterns, reduce training time, and enhance accuracy will be a key area of development in the future.
In addition to performance enhancements, addressing ethical concerns and limitations will play a significant role in the advancement of generative AI models.
As models become more sophisticated and versatile, it will be crucial to ensure responsible use, transparency, and accountability, paving the way for a more ethical and effective future for generative AI.
Conclusion
Generative AI has come a long way since its inception, showing immense potential in various industries and applications. As we continue to develop more advanced models and tools, the opportunities for creative content generation, synthetic data creation, and problem-solving will grow exponentially.
By navigating the challenges, addressing ethical concerns, and embracing the future advancements in generative AI, we can unlock new possibilities and reshape the way we live and work. The future of generative AI is indeed promising, and it’s up to us to harness its full potential responsibly.
Frequently Asked Questions
Is GPT a generative AI?
Yes, GPT is a generative AI. Developed by OpenAI, GPT stands for Generative Pre-trained Transformer and is a type of large language model (LLM). It uses the transformer architecture and is a key advancement in artificial intelligence (AI) powering generative AI applications such as ChatGPT.
What is generative AI vs AI?
Generative AI and Traditional AI both use machine learning algorithms, but have different goals. Generative AI focuses on creating new data and content, while Traditional AI solves predefined tasks with specific rules. Generative AI uses unsupervised models, while Traditional AI typically employs supervised models.
What is the most used generative AI?
Of these 10 generative AI tools, Jasper is the most widely used due to its user-friendly interface and range of features. Click here to find the best AI tools and compare.
How do generative AI models work?
Generative AI models use neural networks to recognize patterns in data and then create something new, be it text, images, audio, or video. It uses algorithms to produce outputs that are statistically probable.
What are the challenges of generative AI?
Generative AI presents a range of challenges, from addressing ethical and bias-related considerations to overcoming resource, technology and stakeholder issues. It’s vital to carefully navigate these obstacles to ensure successful implementations.
Discover More AI Tools
Every week, we introduce new AI tools and discuss news about artificial intelligence.
To discover new AI tools and stay up to date with newest tools available, click the button.
To subscribe to the newsletter and receive updates on AI, as well as a full list of 300+ AI tools, click here.